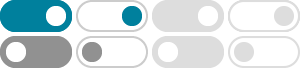
Fully convolutional Deep Stacked Denoising Sparse Auto encoder …
Oct 1, 2022 · The features extracted from the FCN is batched and taken as an input to the DS-DSA and processed to perform feature extraction to reconstruct the partial face from the gallery image. This manuscript proposes a Deep stacked Denoising Sparse autoencoder for removing the error amid the input and output data.
Feature Selection for Imbalanced Data with Deep Sparse Autoencoders ...
Mar 22, 2021 · To achieve FS advantages in this setting, we propose a filtering FS algorithm ranking feature importance on the basis of the Reconstruction Error of a Deep Sparse AutoEncoders Ensemble (DSAEE). We use each DSAE trained only on majority class to reconstruct both classes.
Face recognition via Deep Stacked Denoising Sparse
Aug 15, 2019 · In the proposed Deep Stacked Denoising Sparse Autoencoders (DSDSA) system, a deep network was built to learn the feature representation of face images by automatic facial feature extraction. To reach a robust system two classifiers were used, multiclass-SVM and Softmax classifier.
Network Intrusion Detection System using Feature Extraction …
A deep sparse autoencoder is used to extract features from a typical unsupervised deep learning autoencoder model classified by the Random Forest (RF) classification algorithm. Improvements to the classification performance and detection speed are confirmed.
Deep Sparse Autoencoder for Feature Extraction and Diagnosis …
Jul 15, 2018 · This paper proposes a sparse autoencoder deep neural network with dropout to diagnose the wheel-rail adhesion state of a locomotive. This deep neural network can significantly reduce the adverse effect of overfitting, making the learned features more conducive to classification and identification.
Machine Learning-Based Feature Extraction and Selection - MDPI
Jul 27, 2024 · To this end, authors propose a methodology based on a geographical information system (GIS) to accurately obtain and interpret spatial user location, utilising a deep sparse autoencoder (DSA) to perform feature extraction and a bidirectional optimised hybrid model (BLSTM-DNN-ASOA) to conduct the classification task.
Training a deep network for feature extraction and classification includes unsupervised pretraining and supervised fine-tuning. We utilized stacked denoise autoencoder (SDAE) method to pretrain the network, which is robust to noise.
Stacked Denoise Autoencoder Based Feature Extraction and Classification …
Training a deep network for feature extraction and classification includes unsupervised pretraining and supervised fine-tuning. We utilized stacked denoise autoencoder (SDAE) method to pretrain the network, which is robust to noise.
Effective Feature Extraction via Stacked Sparse Autoencoder …
Jul 23, 2018 · So, in this paper, we propose using the stacked sparse autoencoder (SSAE), an instance of a deep learning strategy, to extract high-level feature representations of intrusive behavior information. The original classification features are introduced into SSAE to learn the deep sparse features automatically for the first time.
In this work, we propose a novel discriminative autoencoder. Use of supervised discriminative learning ensures that the learned representation is robust to variations commonly encountered in image datasets.
- Some results have been removed