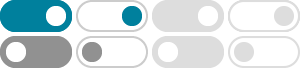
Multimodal Deep Autoencoder for Human Pose Recovery
In this paper, we propose a novel pose recovery method using non-linear mapping with multi-layered deep neural network. It is based on feature extraction with multimodal fusion and back-propagation deep learning.
We train the (b) bimodal deep autoencoder in a denoising fashion, using an augmented dataset with examples that require the network to reconstruct both modalities given only one.
[2205.14204] Multimodal Masked Autoencoders Learn …
May 27, 2022 · We propose a simple and scalable network architecture, the Multimodal Masked Autoencoder (M3AE), which learns a unified encoder for both vision and language data via masked token prediction.
nique for handling missing multimodal data using a specialized denoising autoencoder: the Multimodal Autoencoder (MMAE). Empirical results from over 200 participants and 5500 days of data demonstrate that the MMAE is able to predict the feature values from multiple missing modalities more accurately than
Variational Mixture-of-Experts Autoencoders for Multi-Modal Deep ...
Nov 8, 2019 · Here, we propose a mixture-of-experts multimodal variational autoencoder (MMVAE) to learn generative models on different sets of modalities, including a challenging image-language dataset, and demonstrate its ability to satisfy all four criteria, both qualitatively and quantitatively.
Multimodal Deep Generative Models for Trajectory Prediction: A ...
In this work, we provide a self-contained tutorial on a conditional variational autoencoder (CVAE) approach to human behavior prediction which, at its core, can produce a multimodal probability distribution over future human trajectories conditioned …
[2008.03880] Multimodal Deep Generative Models for Trajectory ...
Aug 10, 2020 · In this work, we provide a self-contained tutorial on a conditional variational autoencoder (CVAE) approach to human behavior prediction which, at its core, can produce a multimodal probability distribution over future human trajectories conditioned on past interactions and candidate robot future actions.
Code supporting the paper "Multimodal Autoencoder: A Deep …
The MultimodalAutoencoder (MMAE) is designed to deal with data in which large, contiguous blocks of features go missing at once; specifically all the features extracted from the same data source or modality.
In this paper we address this problem, propos-ing a system based on multimodal deep au-toencoders that enables a robot to learn how to navigate by observing a dataset of sensor in-put and motor commands collected while being teleoperated by a human.
Deep Auto-Encoders With Sequential Learning for Multimodal …
To address these challenges, in this paper, we propose a novel deep neural network architecture consisting of a two-stream auto-encoder and a long short term memory for effectively integrating visual and audio signal streams for emotion recognition.
- Some results have been removed